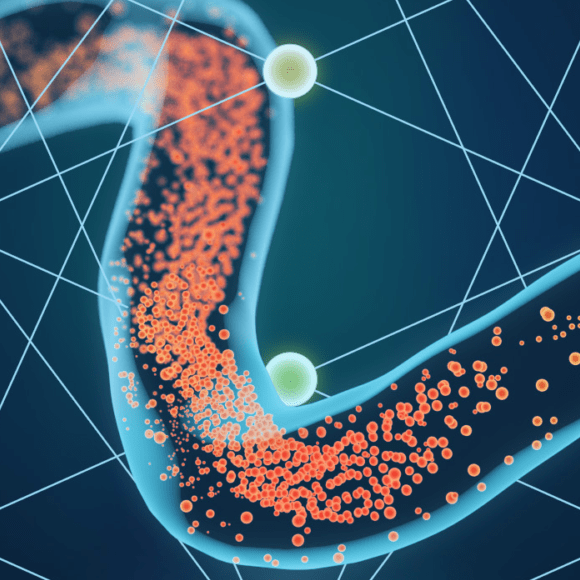
We invent optical and computational tools to improve fluorescence microscopy. We are passionate about applying our tools to problems of outstanding biological significance.
The last few decades have seen remarkable progress in imaging technology. Modern fluorescence microscopy offers the ability to watch biological structure and function with molecular contrast and specificity over diverse spatiotemporal scales. The relatively recent field of super-resolution microscopy has improved spatial resolution to the point that nanoscale protein distributions can be visualized and tracked in living cells. Light-sheet microscopy permits gentle, long-term imaging of cellular dynamics in transparent tissue, while multiphoton microscopy can enable high-resolution imaging of calcium activity even through an intact skull.
Our current work continues to push the boundaries of fluorescence imaging, particularly in living samples. We recently showed that structured illumination, confocal microscopy, multiview imaging, and deep learning synergize to improve fluorescence imaging in densely labeled tissue1. We are building an improved version of this imaging platform and are excited about the prospects of applying it to interrogate 4D Cellular Physiology.
A second project aims to improve adaptive optics (AO) correction of sample-induced aberrations so that this capability may be easily integrated into fluorescence microscopes. While AO has great potential, existing methods are expensive, inefficient, and difficult to implement. These limitations hinder widespread adoption by biologists. With Patrick La Riviere, we have conceived a new method that promises to dramatically simplify AO and are currently in the process of prototyping it.
Deep learning has emerged as one of the most exciting advances in recent years. Inspired by the work of many others, we have developed methods to denoise and deblur raw image data2,3 alleviating tradeoffs between resolution, speed, SNR, and photodamage. Nevertheless, deep learning methods require more validation before they are accepted and broadly used by biologists. While it is often asserted that “seeing is believing”, it might be better to ask, how can we believe what we see? How much can spatial resolution or SNR be improved for a given training set? How linear are the results? Can networks generalize across microscope types, given the known physical and noise characteristics of the image formation process? How much (or little) training data is required for a given application? We are interested in collaborating with machine learning experts to explore these questions.
Finally, although technology development will always be a key focus, we also collaborate extensively with biologists to road-test, break, and refine our methods – and (most importantly) to ensure they enable new biological insight. We’re currently very excited about a collaboration with Daniel Colón-Ramos and Zhirong Bao, attempting to create the first 4D atlas of cell positions, dynamics, and outgrowths in the worm embryo4,5. Eventually, we hope to integrate activity6, behavior7, gene expression, and ultrastructure into our atlas, thereby documenting the 4D wiring decisions of nervous system assembly8 in the full physiological context of the developing organism. We are keen to leverage core Janelia strengths in computation and electron microscopy in pursuit of this goal.
1 Wu, Y. et al. Multiview confocal super-resolution microscopy. Nature 600, 279-284 (2021). https://doi.org:https://doi.org/10.1101/2021.05.21.445200
2 Chen, J. et al. Three-dimensional residual channel attention networks denoise and sharpen fluorescence microscopy image volumes. Nature Methods 18, 678-687 (2021). https://doi.org:https://doi.org/10.1101/2020.08.27.270439
3 Li, Y. et al. Incorporating the image formation into deep learning improves network performance. bioRxiv (2022).
4 Santella, A. et al. WormGUIDES: an interactive single cell developmental atlas and tool for collaborative multidimensional data exploration. BMC Bioinformatics 16, 1-9 (2015).
5 Christensen, R. et al. Untwisting the Caenorhabditis elegans embryo. eLife, e10070 (2015).
6 Ardiel, E. L. et al. Visualizing Calcium Flux in Freely Moving Nematode Embryos. Biophys. J. 112, 1975-1983 (2017).
7 Ardiel, E. L. et al. Stereotyped behavioral maturation and rhythmic quiescence in C. elegans embryos. eLife, e76836 (2022).
8 Moyle, M. W. et al. Structural and developmental principles of neuropil assembly in C. elegans. Nature 591, 99-104 (2021).